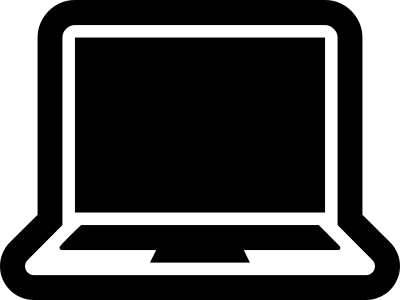
This workshop introduces the topic of text mining and its applications. It covers different encoding mechanisms to convert text into numbers that algorithms can handle. It gives an overview of different text mining tasks, including de-identification, sentiment analysis and document clustering, and how they work with examples and live demos. There will also be references to state-of-the-art tools and libraries to conduct various text mining tasks.
Level: Introductory
Length: 3 Hours
Format: Lecture + Hands-on
Prerequisites: Basic Python
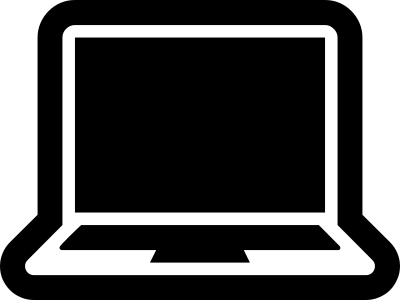
Computational Fluid Dynamics (CFD) is a field of computational physics that has a very high utilization of modern Advanced Research Computing (ARC) resources. The spatial and temporal resolution required to solve modern CFD problems means that it is well suited to take advantage of the full benefits of large-scale distributed memory parallelization that is available on high-performance computing (HPC) systems. As CFD tools have progressed over the past years, their robustness, predictive capabilities, and user-friendliness have drastically improved, which means that these tools are increasingly being adopted by non-traditional HPC users such as new graduate students, experimentalists, theoreticians, and even student design teams.
This course is intended to help learners with a basic understanding of fluid dynamics and CFD bridge the knowledge gap towards the effective utilization of CFD on modern HPC architectures. This course will take an end-user approach to CFD tools on HPC systems (no coding) and, despite some prerequisites, will be given at an introductory/intermediate level (we will not cover advanced topics such as GPU or dynamic load-balancing).
At the end of the course, the learner will be able to:
- Develop a systematic approach to estimate the HPC cost of a CFD problem.
- Explain the impact of modelling assumptions on HPC cost.
- Optimize the parameters and simulations for effective HPC usage.
The course will use an entirely open source suite of CFD toolsets to mesh (Gmsh), simulate (OpenFoam/SU2), and visualize (Visit/Paraview). It should be noted that this is not a CFD course; therefore, undergraduate-level knowledge of CFD and numerical methods is expected, as well as a basic understanding of the Compute Ontario HPC system. The focus is on the effective use of CFD tools in modern HPC systems.
Course structure: The course will be delivered in a hybrid format with three 1.5-hours blocks covering the following topics:
- Planning and estimating HPC requirement (1.5 hours).
- Optimizing CFD simulations for HPC (1.5 hours).
- Running, visualizing, and organizing data (1.5 hours).
The synchronous segments will be used to explain the important theories and concepts and introduce the tutorial problem that the learners will be asked to work on individually between the synchronous sessions. The course is developed based on the newly developed ARC4CFD (https://arc4cfd.github.io) course.
Level: Intermediate
Length: Three 1.5-Hour Sessions (3 Days)
Format: Lecture + Hands-on
Prerequisites:
- Undergraduate-level knowledge of fluid dynamics (ideally with some knowledge of turbulence), CFD, and numerical methods.
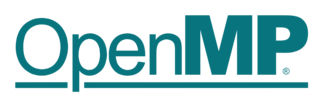
This is an introduction to the intermediate level OpenMP hand-on course. OpenMP is a standard parallel programming API that supports multi-platform shared-memory multiprocessing programming in C, C++, and Fortran.
This one-day course will cover the principles of OpenMP compiler directives, library routines, and environment variables with step-by-step hand-on examples. Case studies include various approaches for loop parallelism. We will also talk about the Task constructs for irregular programs, and the Target constructs for accelerators such as GPU. Participants will have hand-on programming experience with OpenMP as well as how to compile and run Multi-thread OpenMP code on different alliance clusters.
Level: Introductory
Length: Two 3-Hour Sessions
Format: Lecture + Hands-on (Hands-on portion is CPU only.)
Prerequisites: Basic knowledge of C, C++, or Fortran
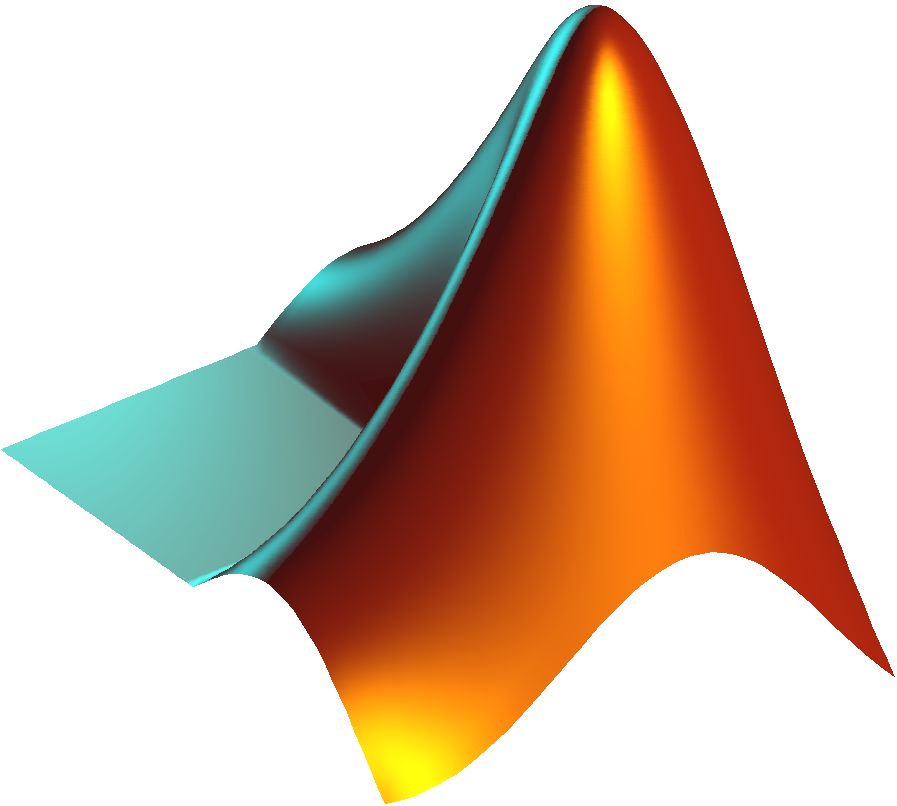
During this hands-on workshop, we will introduce parallel and distributed computing in MATLAB with a focus on speeding up application codes and offloading compute. By working through common scenarios and workflows using hands-on demos, you will gain a detailed understanding of the parallel constructs in MATLAB, their capabilities, and some of the common hurdles that you'll encounter when using them. Users will learn:
- Multithreading vs multiprocessing
- When to use parfor vs parfeval constructs
- Creating data queues for data transfer
- Leveraging NVIDIA GPUs
- Parallelizing Simulink models
- Working with large data
Level: Intermediate
Length: 3 Hours
Format: Lecture + Hands-on
Prerequisites: Working knowledge of MATLAB
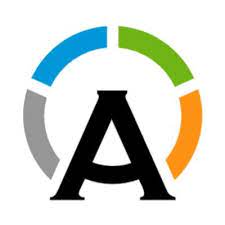
Apptainer is a secure container technology designed to be used on for high performance compute clusters. This workshop will focus on how to use Apptainer as well as how to make use of tools such as Conda and Spack within Apptainer.
By the end of these sessions, one:
- will have learnt about Apptainer and how it is installed and used on our computer clusters,
- how to build an Apptainer container image,
- how to install tools such as Conda/Spack from inside an Apptainer container shell, and,
- how to use Apptainer containers within job submission scripts.
Level: Introductory
Length: Two 3-Hour Sessions
Format: Lecture + Hands-on
Prerequisites: Experience using Alliance compute clusters, e.g., using the BASH shell and submitting jobs.
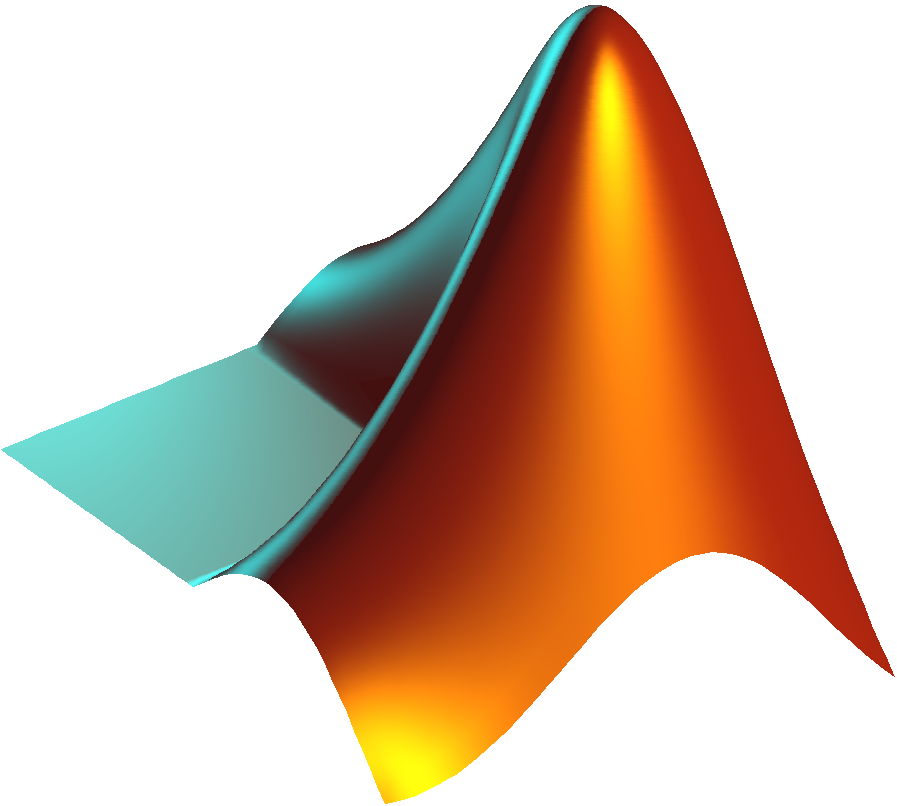
Machine learning is a data analytics technique that teaches computers to do what comes naturally to humans and animals: learn from experience. Machine learning algorithms use computational methods to “learn” information directly from data without relying on a predetermined equation as a model. In this hands-on introductory workshop, you will learn how to apply Machine Learning, and get familiar with the basics of Deep Learning. MATLAB provides an environment to apply advanced techniques without requiring extensive coding nor experience in machine learning.
- Learn the fundamentals of machine learning (supervised learning, feature extraction, and hyperparameter tuning)
- Explore pre-processing and powerful visualization techniques
- Build and evaluate machine learning models for classification and regression of various data formats (signals, images, text)
- Perform hyperparameter tuning and feature selection to optimize model performance
- Discuss interoperability with other platforms
- Learn how to deploy Machine Learning models
Level: Intermediate
Length: 3 Hours
Format: Lecture + Hands-on
Prerequisites: Working knowledge of MATLAB