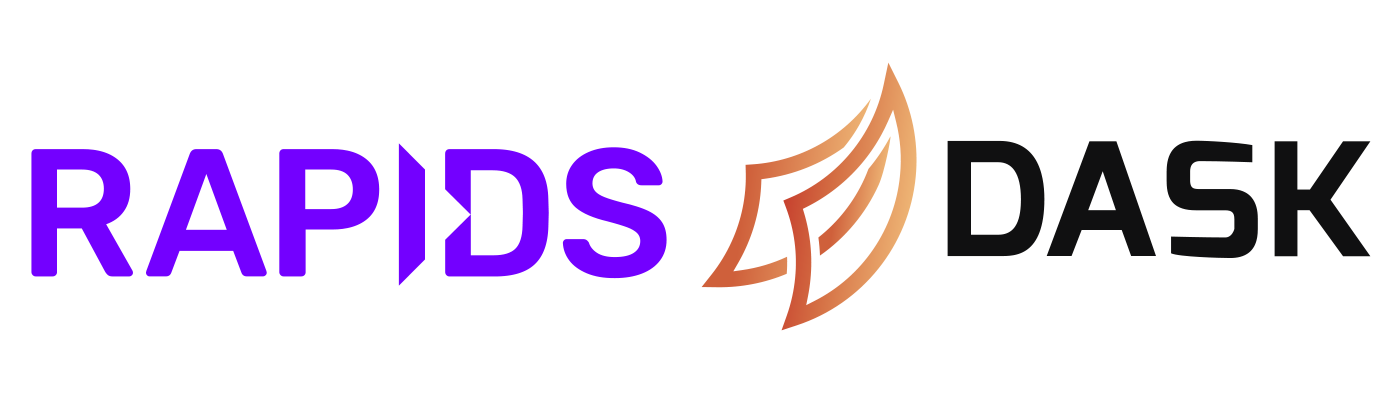
Some popular Python libraries for data analytics, like Numpy, Pandas, Scikit-Learn, etc., usually work well if the dataset fits into the RAM on a single machine. When dealing with large datasets, it could be a challenge to work around memory constraints. This course introduces scalable and accelerated data analytics with Dask and RAPIDS. Dask provides a framework and libraries that can handle large datasets on a single multi-core machine or across multiple machines on a cluster. RAPIDS, on the other hand, can accelerate your data analytics by offloading analytics workloads to GPUs with less effort in code changes.
Level: Introductory
Length: Two 3-Hour Sessions (2 Days)
Format: Lecture + Hands-on
Prerequisites:
- Alliance Account
- Basic Python and Linux command line experience.
- Teacher: Jinhui Qin
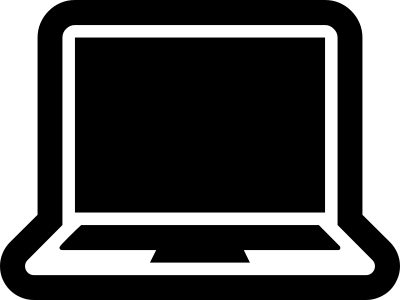
Have you ever tried to run someone else’s code and it just didn’t work? Have you ever been lost interpreting your colleague’s data? This hands-on session will provide researchers with tools and techniques to make their research process more transparent and reusable in remote computing environments. You’ll be using platforms like JupyterHub and command-line tools like Bash and Docker in a Linux environment to interact with the material through various exercises and examples.
In this workshop, you’ll learn about:
- organizing your file directories
- writing readable metadata with README files
- automating your workflow with scripts
- capture and share your computational environment
Level: Introductory
Length: 3 hours
Format: Lecture + Hands-on
Prerequisites: Initial familiarity with command line tools and/or a Linux environment may be beneficial but not mandatory
- Teacher: Sarah Huber
- Teacher: Shahira Khair
- Teacher: Drew Leske
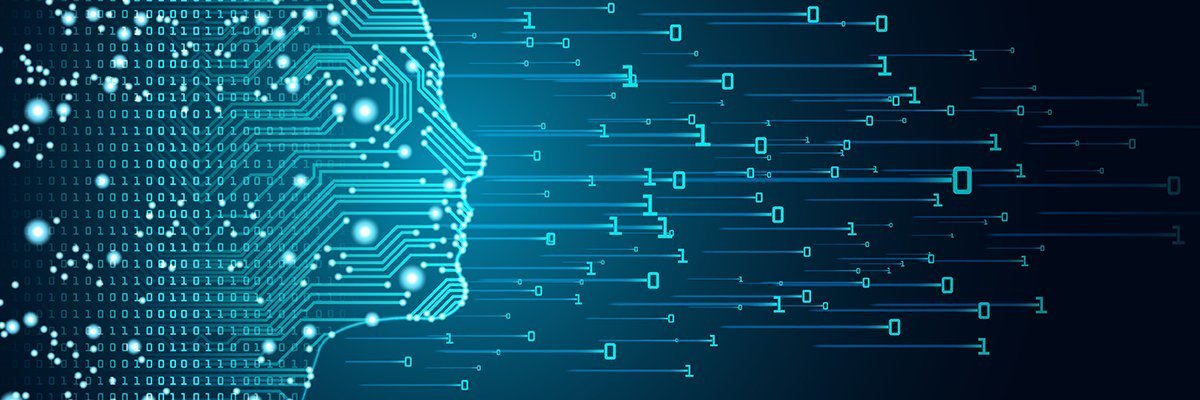
This course provides an introduction to machine learning that enables computers to learn AI models from data without being explicitly programmed. It comprises two parts:
- Part I covers the fundamentals of machine learning, and,
- Part II demonstrates the applications of various machine methods in solving a real world problem.
Rather than presenting the key concepts and components of machine learning in an abstract way, this course introduces them with a small number of examples. By using plotting and animations, insight into some of the mechanics of machine learning can be had. Furthermore, the student will gain practical skills in a case study, in which each step of developing a machine learning project is presented. By the end of this course, the student will have a solid understanding and experience with some of the fundamentals of machine learning enabling subsequent exploration.
Level: Introductory to Intermediate
Length: Two 3-Hour Sessions
Format: Lecture + Hands-on
Prerequisites:
- Data preparation or equivalent knowledge.
- Basic Python knowledge and experience.
- Knowledge and experience with Tensorflow and Scikit-learn would also be helpful.
- Teacher: Weiguang Guan
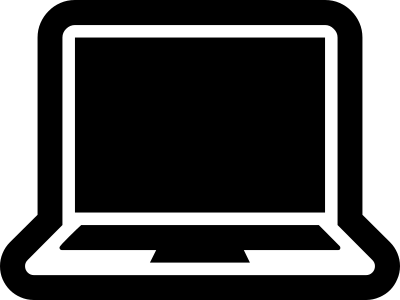
NOTE: This course is divided into four (4) parts over three (3) days.
Part I and Part II Description:
Introduction of neural network programming concepts, theory, and techniques. The class material will begin at an introductory level, intended for those with no experience with neural networks, eventually covering intermediate concepts. (The Keras neural network framework will be used for neural network programming but no experience with Keras will be expected.)
Part III Description:
This part will continue the development of neural network programming approaches from Parts I and II. This part will focus on generative methods used to create images: variational auto-encoders, generative adversarial networks, and diffusion networks.
Part IV Description:
This part will continue the development of neural network programming approaches from Parts I through III. This part will focus on methods used to generate sequences: LSTM networks, sequence-to-sequence networks, and transformers.
Level: Intermediate
Length: Four 3-Hour Sessions (3 Days)
Format: Lecture + Hands-on
Prerequisites:
- Experience with Python (version 3.10) is assumed.
- Each part assumes what was covered in the previous parts of this course.
- Parts III and IV assume experience with neural network programming, per the first two neural network programming sessions in this course.
- Teacher: Erik Spence
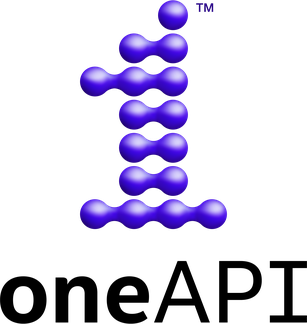
oneAPI is a unified application programming interface intended to be used across different compute accelerator architectures, including CPUs, GPUs and AI accelerators. It's aim is to unify the programming model as well as simplifying cross-architecture development. It also provides libraries for:
- deep neural network (DNN) learning applications,
- collective communications for machine learning and deep learning projects, and,
- data analytics making big data analysis faster using optimized algorithms.
By the end of this workshop one will have:
- learned about oneAPI libraries and the inference toolkit, components, and capabilities for developing and deploying computer vision and deep learning solutions,
- explored techniques for optimizing pre-trained deep learning models and learn how to work with models from different frameworks like Tensorflow, PyTorch and Caffe,
- understood how to perform inference on different hardware such as CPU and GPU, and,
- considered practical computer vision applications and use cases.
Level: Intermediate
Length: 3 Hours
Format: Lecture + Hands-on
Prerequisites:
- Attendees having hands-on experience with Python and some experience with Tensorflow or PyTorch will get the most out of this workshop.
- Teacher: Peter Darveau